Table of Contents
In the contemporary digital world, it is no surprise that the field of Big Data Analytics (engineering, analysis, science, etc.) is booming at a rapid pace. Due to this fact, more and more engineers and analysts are attracted to it and are seeking knowledge and wisdom regarding how to become a data engineer, a data scientist, a data analyst, etc.
I can relate to all these people when I say that when you consider entering the field of Big Data, you always wonder about what are the use cases of Big Data. Specifically, what are the industries where Big Data is used, and what are its applications?
At the start of your career, you might work in a few particular domains, depending on the company you are working in. Because of this, exposure to other industries becomes slower. To solve this, I have mentioned some of the most common industries where big data is used and its applications as well. In this way, you can get an overall idea of how things go in other industries and domains.
As science and technology progress, all sectors are becoming digitized (if not already). And with this and the advent of the cloud, industries are scaling their businesses, which, in turn, is resulting in the proliferation of data.
With such widespread big data, industries are looking for ways to optimize and improve their business processes and operations, get a 360° view of their customers, get ahead of the competition, and achieve cost-effective results. In short, Big Data helps industries in:
- Optimizing assets & resources
- Identifying potential bottlenecks
- Decreasing inefficiencies & increasing productivity
- Reducing costs & increasing ROI/Sales
Industries Where Big Data is Used
Today, I’ll go through the top 5 industries where big data is used and give you a big picture of what’s possible with the power of data analytics, what are some of the use cases of big data, and what problems Big Data solves.
1 Big Data in Finance Industry
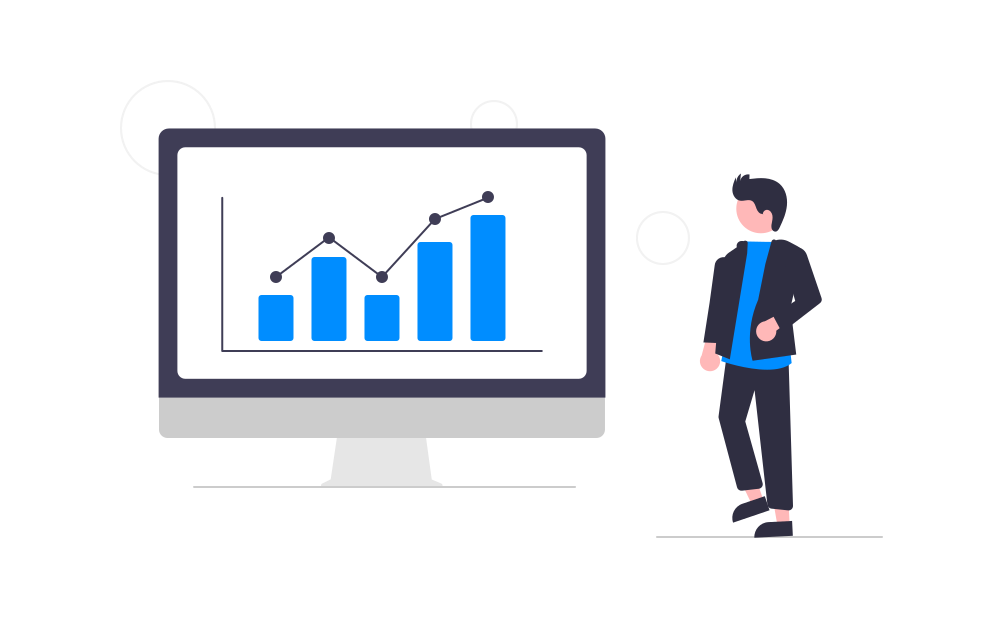
One of the most data-intensive industries out there is the Finance industry. Data is the backbone whether it’s banks, stock exchanges, or fintech companies. The finance industry generates a huge amount of data. Be it in the form of monetary transactions or real-time trends in the stock exchange. And to use and utilize this data is a responsibility that financial institutions need to bear.
Big data helps financial institutions mitigate risks, increase ROI in different investments, identify potential harms, detect frauds proactively, etc.
Below are some of the use cases of big data in the finance industry:
- Customer Segmentation & Relationship Management: The finance industry runs when there are customers. For these industries to retain customers, it is paramount for them to retain these customers and provide proper value. For this reason, big data can be utilized. Big data helps organizations in segmenting and categorizing customers, with the help of which, they can target these customers with customized products or solutions, identify the segment of customers who are interested in any specific product or service, and recommend new products or services which are of most interest to this segment. This helps increase revenue and sales and improves customer experience. Not only that, organizations can market specific customer segments with customized products just for them. They can cut costs by only marketing the relevant segments, and hence, generating more ROI. In the world of organizations being competitive, it is paramount to retain customers, and big data is the obvious product that helps organizations achieve this feat.
- Financial Planning: So why is financial planning important? Companies do financial planning to reduce any potential risks or hurdles in their financial goals. Big data is a big asset to help companies in this regard. Also, big data can be used by a financial planner who might use this data to help a client or customer understand the likely future performance of different investment options. He can predict the right course of action and based on probability, can predict the right roadmap to follow. And since this would be factual and backed by data, it is more likely to be proven in favor of the client in the long term.
- Digitization of Modern Banks: Banking is rapidly becoming digital. More and more banks are being opened which are online and rarely have any physical branches. For this to happen, rapidly growing technology has a big role to play. For banks to be operational, one of the aspects is the operational data, based on which, several internal digital products perform their intended operations. For example, Operational Data Stores (or ODS) are being developed. ODS is a repository of operational data gathered from multiple sources in real-time to create digital products. For example, banks can use ODS as a backend for their CRM systems for real-time customer service. Banks can also develop sophisticated and intelligent systems based on AI by using big data, which can detect potential scammers and phishers who have malignant intentions. Other than that, digital banks can increase their efficiency. They can optimize the workflow and user experience by analyzing the big data related to this. This can exponentially increase the customer experience in a positive sense. Also, banks can use big data to detect fraudulent activities and transactions, and in doing so, mitigate the financial risks involved in it.
- Predictive Analytics: By using data and the power of artificial intelligence, financial institutions can use predictive analytics to solve various business problems. Following are some of the common ones:
- Stock Exchange Trends Forecasting
- Customer Churn Prediction
- Product Performance Prediction
- Workflow Optimization
- Identification of high-value customers
2 Big Data in Manufacturing
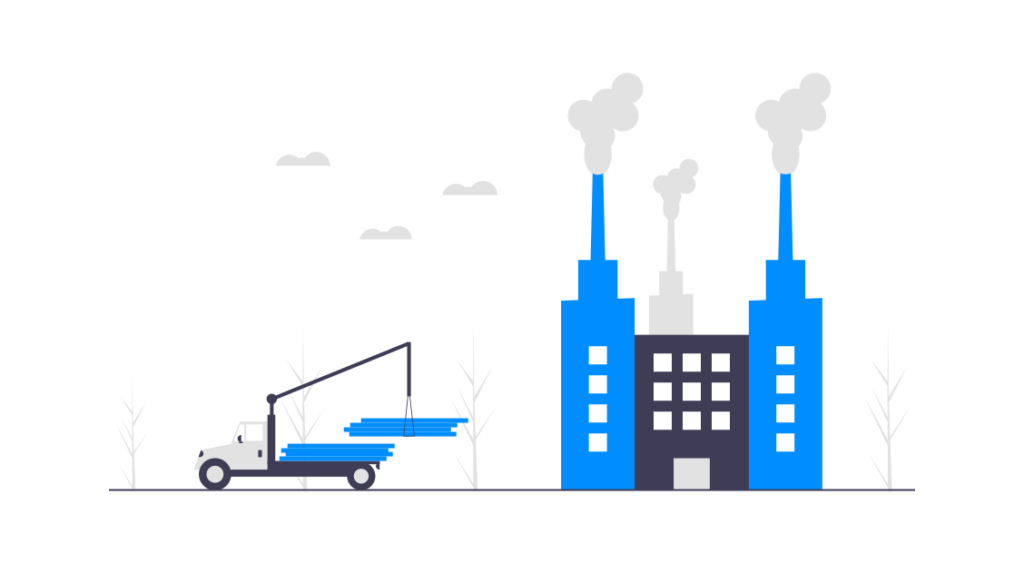
Manufacturing is on the verge of using and applying data and advanced analytics. Manufacturing is a broad term, which encompasses many domains, be it the automotive industry, electronics manufacturing, aerospace, or general manufacturing of several kinds of machinery used in different domains.
Now, sophisticated machinery is used in manufacturing. For example, robotic arms for assembling and packaging products, different kinds of sensors to sense any potential anomalies, etc.
The data is collected from these sensors, robots, cameras, and machinery, based on which, important decisions can be made. Decisions like:
- Optimizing the workflow of a factory
- Improving production processes
- Cost reduction & optimization
- Improved supply chain management
- Reducing carbon footprint
- Increased Automation
A large amount of data is collected from different sensors, data like temperature, humidity, pressure, and other parameters responsible for maintaining the health of the products being manufactured. By analyzing this data, several inefficiencies and bottlenecks can be identified, which can later be minimized (or removed). This data can also be used to train ML models for predictive analytics and machine learning which ultimately helps in forecasting the demand and supply for their products and adjusting the production in a way that generates more ROI and is more cost-effective.
Let’s discuss some of the points.
- AI in Manufacturing: I have touched on machine learning above but in simple terms, We can use machine learning for Predictive Analytics, which means, we can predict patterns responsible for various anomalies which can help us mitigate them, and patterns responsible for affecting customer value or increasing ROI. Prescriptive Analysis can help us identify the factors which, when acted upon, have the potential to exponentially increase productivity as well as set optimal conditions for maximum ROI. Not only that, Image Recognition technology can be exponentially helpful in tagging products and goods, as well as the security of the products & packaging.
- Optimization & Reduced Cost: Manufacturing usually requires complex processes. And complex processes often result in unmonitored costs. This cost can be reduced and the overall process can be optimized provided the right data analytics ecosystem is in place and KPIs are analyzed properly.
- Carbon Footprint Reduction: The supply chain is a major thing in manufacturing. Especially, in the world of E-Commerce where people purchase several goods online. Online stores receive those goods from their respective manufacturers and then, ultimately, send these goods to the customers. All of this takes the supply chain to work. And this, in turn, potentially causes carbon emissions which is a big environmental hazard. Now, to reduce the Carbon Footprint, Big Data can be used to analyze the Carbon Footprint data. However, collecting, storing, and processing this data is a challenge with different industry leaders trying to solve it.
- Post-COVID-19 Effect on Manufacturing: COVID-19 lockdown significantly affected the manufacturing industry. Although the lockdown is over in most countries, it accelerated the digitization of industries, including the manufacturing industry. In doing so, it forced industry leaders to think about optimizing the supply chain process. Be it in the form of enforcing to implement policies related to the safety of workers more efficiently, or in the optimization of transportation of manufactured goods, Big Data Analytics is playing a massive role.
The adoption and use of big data analytics in the manufacturing industry are still in their early stages & it will take some time to get mature.
3 Big Data in Healthcare
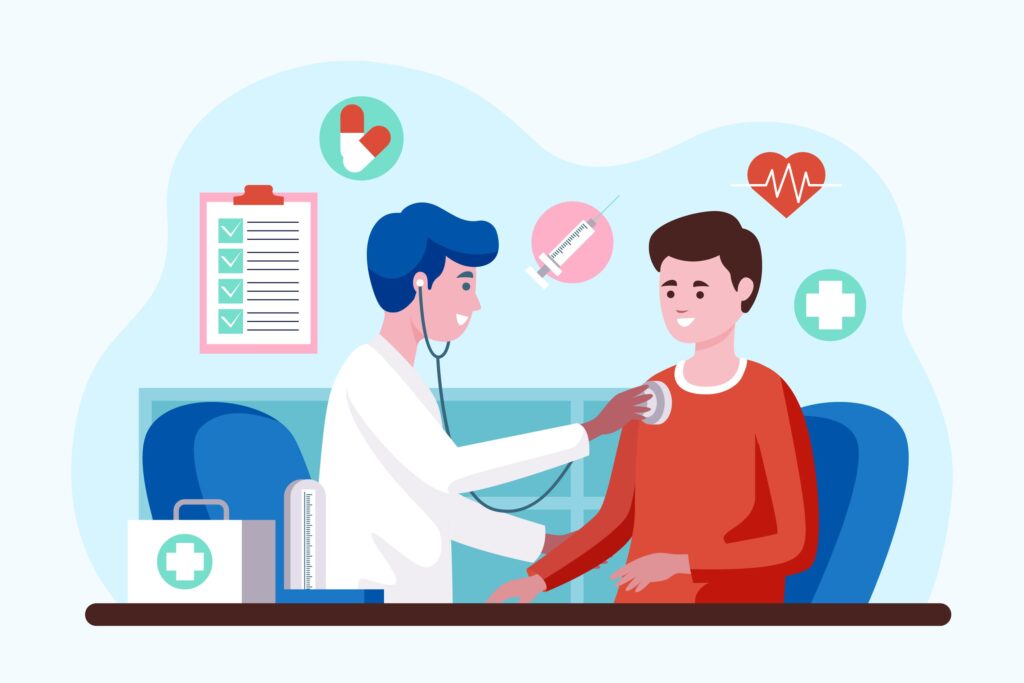
Healthcare is one of the major industries which is revolutionized by technology and Big Data Analytics. From aiding medical professionals in performing their tasks efficiently to detecting cancerous cells in the human body using machine learning, Big Data has come a long way.
To a human being, healthcare is of utmost importance in his/her life. With the help of Big Data Analytics, healthcare can potentially be made more efficient in terms of the health and safety of people. It can help HCPs (or Healthcare Professionals) perform their jobs more efficiently.
One example of how big data is being used in healthcare is through the analysis of electronic medical records (EMRs) to identify patterns and trends that can inform the development of more effective treatment strategies. Big data analytics can also be used to identify risk factors for certain diseases and to predict patient outcomes.
Let’s discuss how exactly Big Data Analytics aids healthcare:
- Less Error-Prone Identification & Prediction of Diseases: Sophisticated machine learning algorithms can be trained on Big Data to identify and predict diseases before they occur. Active research has been going on on different fatal diseases to cure them (or at least, predict them). For example, different scientists are using Big Data Analytics to research predicting cancer cells in the body. Scientists also make use of genomics data for further research.
- Personalized Medicine & Treatment: By looking at the medical history of patients, which is enabled by the availability of electronic medical records (EMRs), doctors can predict certain trends. With the help of these trends, doctors and other related HCPs can formulate a better treatment. In fact, AI systems can help create treatment plans automatically which the doctors and medical professionals can tweak as per the need of the specific patients.
- Identification of Potential Risks: Big Data Analytics can help in the identification of potential risks. For example, risks involved in the treatment of a patient, environmental hazards, the health of medical staff, etc. KPIs can be made and visualized over dashboards for HCPs to monitor problems and then come up with solutions to those problems. This makes the whole healthcare process smoother, more optimized, and less error-prone.
- Public Health Trends: Due to the availability of electronic health records or EHRs, and their anonymous availability online, this data is rich and extremely helpful for analysts to analyze this data and discover undiscovered problems and their potential solutions.
In essence, healthcare is one of those industries where Big Data is tremendously used and has revolutionized healthcare in an impactful way. By gathering and analyzing large datasets, healthcare providers can make smarter decisions in diagnosing and treating patients.
Big data can also bring together information from multiple sources (such as lab results and medical records) to provide clinicians with a more detailed picture of the health status of their patients. Harnessing big data’s potential is essential for helping healthcare organizations achieve quality patient care outcomes while providing cost savings at the same time.
4 Big Data in Telecommunications
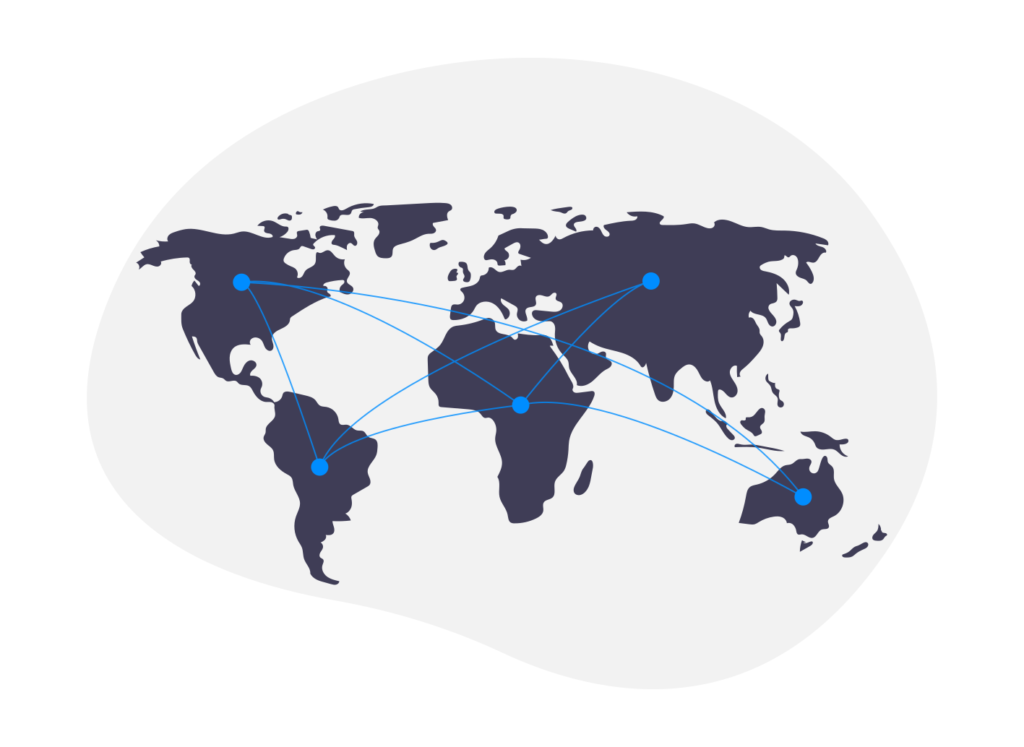
One of the major industries where big data is used is the telecommunication industry. The telecommunications industry is huge. What this industry does is make communication on a global scale (be it national or international) possible.
The telecommunication industry is responsible for the physical infrastructure which includes towers, cables, signals going through airwaves, etc. The biggest companies in this sector are telephone or network operator companies. In Pakistan, companies like PTCL, Jazz, Telenor, etc. are all telecommunication (or telecom) companies. Internationally, some of the big sharks in this industry are T-Mobile US Inc, Verizon, AT&T, etc.
This sector generates a humongous amount of data. Telecommunication companies are usually big corporates with a lot of moving pieces. So data is generated not only from the technical side of things but organizational data is also often big. Some example of data generated is as follows:
Call Data Records (aka CDRs) | These records contain information about calls made between customers. Information like phone numbers, duration of calls, location of the devices, the time at which the call started, etc. This data is huge considering calls are happening all the time in the country. And managing and processing this data becomes quite challenging. Sophisticated big data systems are put in place to process & store this data. |
Text Messaging Data | These records contain information regarding text messages being sent between customers. The information contains the data regarding the time messages were sent/received, who were the recipients, the phone numbers of both senders and recipients, etc. |
Internet Usage Data | Although it might come off as scary, telecom companies collect internet usage data as well. Data can be regarding the websites visited, the amount of data transferred by customers on those websites, the visit of customers to any competitor’s website, etc. |
Network Data | This includes data about the performance of the telecom network, such as signal strength and network congestion. |
Customer Data | This one is so obvious, these companies collect their customers’ data to perform several forms of analytics on them. This data can be the general identification of customers, their financial spending on products, and payment history and patterns. |
The above table shows the data which is usually collected by these companies. However, this may vary from company to company.
Now, the question is: how is this Big Data used in this industry? Following are some of the use cases of Big Data in the telecom industry:
- Fraud Detection: For these companies to maintain the highest quality, they need to retain their customers. For this, these companies ought to put in place the best security practices. These companies use different kinds of data to detect fraud. These fraudulent activities can be any unauthorized use of any product or service, identity theft, wrongful use of credit/debit cards, etc.
- Customer Segmentation: Big Data can be used on algorithms like K-Means Clustering to segment customers into different categories. In this way, companies can target each particular segment based on their specific pattern with marketing SMS and things like that. You may have noticed that if you and your friend are on the same network but receiving different marketing messages through SMS service, this would likely mean that, based on both of your patterns, you both may lie in different segments. Customer segmentation is really helpful as it helps telecom companies optimize their marketing campaigns and reduce costs in the long run.
- Network Optimization: Telecom companies can use the data coming from cell towers and analyze it to identify and predict potential bottlenecks. These companies can use this data to optimize their network performance and, ultimately, maintain the quality of their products and services. Network optimization is important when multiple telecom companies are competing with each other. The better the performance of the company, the more userbase that company can obtain.
- Marketing & Advertisement: I have touched on this in the Customer Segmentation section above. But I wanted to mention it separately too because I wanted to put more emphasis on it. The telecom industry uses big data for marketing and advertising. They can predict their customers’ patterns and strategize their advertisements based on these patterns. In this way, these company not only save their costs but can make advertisements that are more effective and result-driven.
- Personalization of Products & Services: By analyzing big data, telecom companies can personalize their products and services based on the profiles of their customers. These companies can personalize their products based on the trends their customers are showing. Customer Segmentation is one such factor that helps these companies personalize their products for specific segments of their customers.
Not only that, but these companies also make use of Big Data for several benefits, including measuring their internal KPIs. They can create these KPIs to measure the performance of their employees or optimize their internal workflows for better and more efficient results. Hence, usually, most of these companies maintain their own Data Warehouses.
Also, we have seen a trend here in Pakistan that telecom companies are stepping into the world of digital banking. Launching products like EasyPaisa, JazzCash, etc. They can now easily market their banking products to the massive data of customers they have.
In essence, in this industry, big data is used in a very valuable way. These businesses majorly rely on data to run and that makes big data one of the most important assets of the current world.
5 Big Data in E-Commerce
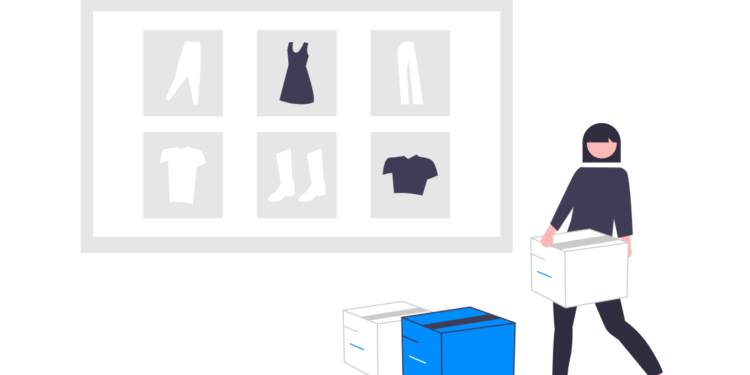
As the internet started to take off in the late 80s, and then fast forward to the mid-90s, we saw a proliferation of personal computers. World Wide Web or WWW provided general public access to information online. This was Web 1.0.
Then came Web 2.0 where the general public could put their own content on the web. Webpages were made interactive as opposed to before when webpages were mostly static. In this era, it was a no-brainer for people and companies to consider selling online. The Internet provided a platform for people to be able to sell and purchase goods on the web. Websites like Amazon, Alibaba, etc. started to rise in this space which gain popularity really fast.
And now, here we are. We see that these E-Commerce companies generate a huge amount of data. And not analyzing this data to improve and optimize business processes would not be a wise move.
70% of Americans are shopping online. And the number of shoppers has been on the rise in America as per the graph below (See caption for credit):
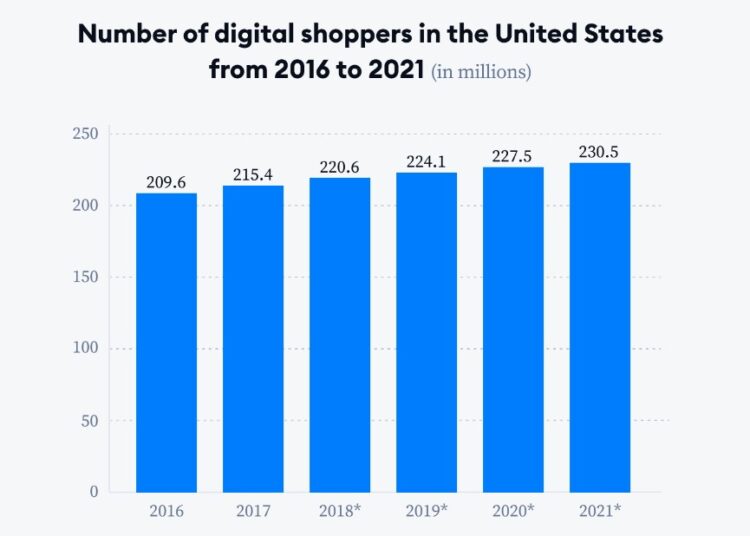
With that said, let’s look at some of the use cases of big data in this industry.
- Customer Service Optimization: With the help of big data analytics, E-Commerce companies can optimize and improve their customer service exponentially. They can analyze the KPIs of their agents to determine their rate of success. They can also analyze the customer pattern to help them with better help needed.
- Product Recommendation: This is, by far, the most common use case. Have you ever wondered while shopping online that the recommendations you are getting are extremely and amazingly relevant to you? That’s because the company you are shopping from has deployed recommendation algorithms that, by using your purchase pattern, are recommending products that are more personalized to you. Isn’t this amazing?
- UX/UI Optimization: Yep, you read that right. This may come as a surprise to some of you but these companies even analyze the data of where customers click more on their website, how much time they stay on the product page, and what certain factors made them stay on or leave the page, etc. By analyzing this data, the engineers can re-design the webpages for maximum ROI. The better the user experience of a customer, the more they are likely to buy from their online store.
- Sentiment Analysis: The reviews that customers leave on the E-Commerce companies’ products, this data can be used to analyze the sentiment of customers by using various AI techniques. E-Commerce companies analyze customer reviews and feedback to improve their offerings. It is paramount for these companies to understand how their customers feel about them. By analyzing their customers’ feedback, they can identify potential areas for improvement and build solid relationships with their customers.
- Increasing Sales: Well, the main reason E-Commerce companies use big data analytics is to ultimately drive more sales and optimize costs. Companies can identify trends in this space and based on this, they can make better and well-informed decisions to create strategies for future sales.
Overall, using big data analytics is extremely important in the E-Commerce space. Especially when multiple competitors are coming to the surface. However, this is a good thing. More competitors mean more competition. And more competition means better quality products for the consumers. And big data surely helps this industry to foster.
Discussion about this post